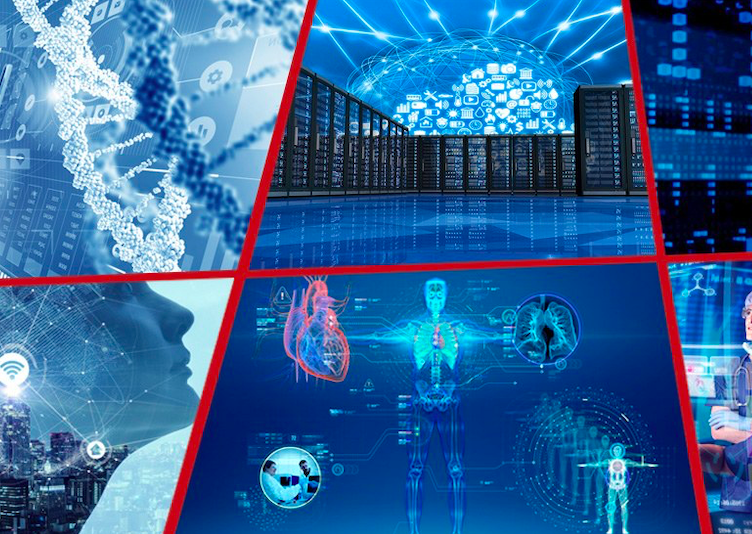
Kick-off workshop (28 April 2021)
Alma Human Artificial Intelligence (AlmaHAI) è un Centro interdisciplinare dell'Università di Bologna, che mira ad aggregare e potenziare le attività di ricerca basate sull’IA presenti in molti Dipartimenti dell’Ateneo, posizionandolo ai primi posti a livello internazionale nella ricerca, nell'istruzione, nell’innovazione e nell’impatto sociale, nonché promovendo un approccio alla ricerca sull'IA da diverse prospettive complementari.
Questa pagina si riferisce al kick-off workshop (28 Aprile 2021) dell'area scientifica AI and Hard Sciences del Centro AlmaHAI, coordinata dal Prof. Antonio Zoccoli. Il workshop è stato organizzato dal Prof. Daniele Bonacorsi e dal Prof. Pierluigi Contucci, in congiunzione ad un analogo kick-off workshop il giorno precedente (27 Aprile 2021) dell'area Foundations of AI l'agenda del quale si trova a questo link e al quale ci si può connettere via Teams. I due workshop sono stati organizzati in giornate adiacenti per sollecitare interesse e partecipazione ad entrambi e stimolare discussioni e possibili sinergie tra vari ambiti di ricerca e aree di interesse.
Segue agenda dettagliata.
Le presentazioni in agenda sono riportate nel seguito. Gli slot delle singole presentazioni sono comprensive di (almeno) 5 minuti per Q&A ciascuna. Non appena disponibili, i link per il download delle slides saranno attivati. Al termine del workshop, sarà possibile scaricare un unico file compresso con tutte le slides, che resterà disponibile su questa pagina. Se non vi saranno obiezioni dai partecipanti, i contributi verranno registrati e le registrazioni verranno rese accessibili su questo sito.
Michela Milano (DISI), Direttrice Centro Alma-HAI
Antonio Zoccoli (DIFA), Presidente INFN
Gli organizzatori
Federico MARULLI (DIFA)
L'enorme quantità di dati oggi disponibili ha drasticamente cambiato il nostro modo di fare scienza. La Cosmologia non è un'eccezione, è anzi una delle branche scientifiche maggiormente rivoluzionate. La ricerca in questo campo rappresenta dunque un contesto ideale in cui testare le più innovative tecniche di analisi dati. Nel mio intervento presenterò nuovi progetti scientifici finalizzati allo studio delle proprietà a grande scala dell'Universo tramite l'utilizzo di Reti Neurali Artificiali. In particolare discuterò di come poter allenare e validare questi algoritmi di apprendimento automatico su cataloghi simulati di galassie e ammassi di galassie. Verranno infine presentati i possibili risultati che potranno essere ottenuti grazie a queste nuove metodologie, in particolare per lo studio delle proprietà dell'Energia Oscura, l'enigmatica forma di energia che reputiamo responsabile dell'espansione accelerata dell'Universo.
Fabrizio LILLO (DIM)
Deep neural networks have shown to be often effective in forecasting time series. However sometimes one is interested in estimating parameters of deterministic or stochastic time series models. In economics and social sciences, for example, parameters of a time series model can be associated with unobservable characteristics of individuals, firms, etc. The estimation of model parameters is challenging when the time series is short or when the model is complex. In this presentation I show some examples of this approach with applications to dynamic models of financial time series. In particular, I will mainly focus on a unimodal map with heteroscedastic noise describing the dynamics of bank leverage and I show how deep neural networks can be used to infer the parameters of the map from short (T=59) time series in order to identify their possible chaotic behavior.
Daniel REMONDINI (DIFA)
AI finds many applications in several applied physics fields, in particular on biological/bioclinical/biomedical grounds involving complex high-dimensionality data, such as imaging and genomics. Different methodologies, involving different Deep Learning architectures, can be grouped under the hat of data processing and embedding. This talk will provide an overview of recent state-of-the art applications developed by our group, ranging from MR fingerprinting and Quantitative Susceptibility Mapping for physical parameter estimation in clinical imaging data, to super-resolution for natural and biomedical image enhancement, and COVID-19 Spike protein sequence NLP analysis to score grammaticality and immunological escape. Some of these applications shed light on fundamental questions related to identification of embedded manifolds, with implications for the AI topic of semi-supervised learning. The topics can be further deepened in more extended talks if requested.
Francesco CAMILLI (DIM)
Boltzmann machines are widely used for machine learning applications although we have little understanding of their statistical behaviour. From the Statistical Mechanics perspective their corresponding direct problem, i.e. the computation of the thermodynamic potentials with typically i.i.d. couplings, is a spin-glass model whose solution can be really challenging. In my talk I will address the direct problem of Boltzmann Machines on the Nishimori line, a subregion of the phase space of a spin-glass where the limiting free energy is proved to fulfill a finite dimensional variational principle. As I will try to explain the Nishimori line also provides a bridge between disordered systems and high dimensional Statistical Inference.
Daniele BONACORSI (DIFA)
Advancing our understanding on the fundamental particles of nature and their interactions - as currently summarized by the Standard Model of Particle Physics - has required massive efforts in the design and construction of experiments that operate at the highest energies and intensities, and that produce extremely large and information-rich data samples. The use of machine learning (at large) techniques is revolutionizing how we handle this data and how we interpret its content, greatly increasing the precision of crucial physics measurements as well as the boosting the discovery potential of present and future experiments. This talk presents a brief overview on challenges and opportunities in exploiting ML/DL techniques in High-Energy Physics, on evolutions in their adoption over time, on the computing challenges to face in large-scale training and ultra-fast inference needs, in order to support a world-wide distributed community of thousands of users doing research at the frontiers of particle physics.
Andrea ASPERTI (DISI)
Variational Autoencoders are a popular technique for generative modeling, particularly appreciated for the strong probabilistic foundation, the fast and tractable sampling and the relatively stable and reliable training. In this talk we briefly introduce the topic, and address the several theoretical and technical problems that still hinder this generative paradigm.
Davide VODOLA (DIFA)
Negli ultimi decenni, grazie anche ai progressi sperimentali nel controllo di sistemi microscopici quali atomi, molecole e ioni, si è avuto un crescente interesse da parte di molte discipline scientifiche nello studio e sviluppo di algoritmi quantistici e in una loro possibile realizzazione sperimentale. Tali algoritmi che operano sui qubit, l'unità di base dell'informazione quantistica, sfruttano le caratteristiche peculiari della meccanica quantistica come la sovrapposizione degli stati e l'entanglement, ed effettuano le operazioni in maniera più efficiente rispetto ai computer classici. Le applicazioni di questi algoritmi sono molteplici e si trovano in svariati ambiti, ad esempio, nei problemi di ottimizzazione, nel machine learning, nella crittografia e nella simulazione di sistemi complessi. La realizzazione sperimentale di tali algoritmi sta anche muovendo i primi passi nei cosiddetti noisy intermediate-scale quantum (NISQ) devices che sono processori quantistici che presentano ancora livelli di rumore relativamente alti e che sono ad oggi formati da poche centinaia di qubit. La maggior parte degli algoritmi che viene implementata sui NISQ è costituita da algoritmi ibridi che affidano la parte difficile del calcolo al processore quantistico ed effettuano una parte trattabile classicamente su un computer classico. L'esempio tipico di tali algoritmi è costituito da algoritmi variazionali, in cui i parametri dell'algoritmo quantistico che contengono la soluzione cercata vengono aggiornati in base ad un processo di ottimizzazione che viene svolto su un computer classico. Le prime proposte di algoritmi variazionali sono il Variational Quantum Eigensolver (VQE) apparse per risolvere problemi di chimica quantistica e il Quantum Approximate Optimization Algorithm (QAOA) per risolvere problemi di ottimizzazione combinatoria. Ad oggi, la maggior parte delle tecniche per effettuare l'ottimizzazione classica dei parametri per il QAOA è svolta tramite metodi di discesa del gradiente. In questo lavoro cerchiamo delle alternative a tali metodi di ottimizzazione e ne implementiamo delle realizzazioni su un processore NISQ. In particolare, ne verifichiamo le prestazioni e le possibilità di successo confrontandole con algoritmi esistenti.
Daniele TANTARI (DIM)
Statistical Mechanics offers a fruitful perspective on several research topics in Machine Learning. I will consider, in an unsupervised learning context, models with many hidden units that play a central and are the basis of Deep Learning. I will show that statistical mechanics allows to characterize the representational power of these models, to study their reliability in extracting features from data, to recognize and understand different phases of their learning process and to develop new training algorithms. I will explore recent advances and possible future research directions.